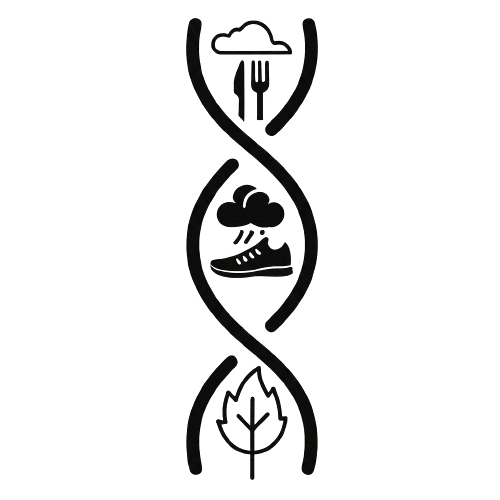
What type of genomic data do you work with?
Genomics encompasses a wide variety of data types that provide comprehensive information about the structure, function, evolution, and mapping of genomes. Some of the key data types we use in the Dennis Lab include whole genome sequencing (WGS), genotype microarrays, and epigenetic markers from DNA methylation microarrays.
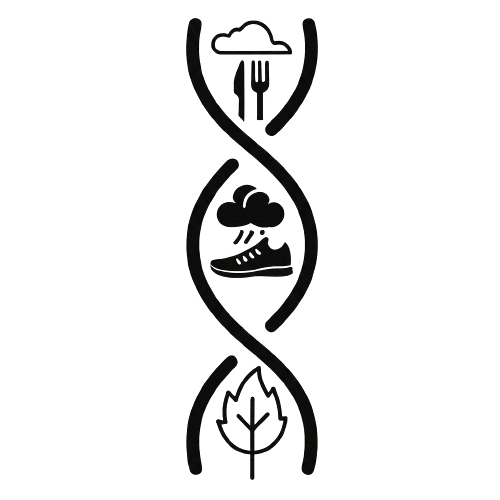
How does genomic data relate to time-varying environments?
While our genotype is fixed at conception, environments change over time, and our genotype may influence how we react to these environments. In the Dennis Lab, we are exploring novel ways to model gene-environment interactions, focusing on how exposures at different life stages contribute to health effects. For example, we are studying how exposure to wildfire smoke in early childhood interacts with genotype to affect lung and brain development, which could ultimately increase the risk of asthma and neurodevelopmental conditions like attention-deficit / hyperactivity disorder (ADHD).
In addition, in contrast to genotype data, other genomic data types are dynamic over space and time. For example, epigenetic markers like DNA methylation vary across cells and tissues, at different stages of the life course, and in response to changing environments. In the Dennis Lab, we study how these genomic data types are regulated in developmental tissues like the placenta, and how this regulation relates to future disease risk. We also study how genomic data types change in response to environmental stimuli, and whether the biological embedding of these stimuli depends on when the stimuli occur relative to sensitive periods of development.
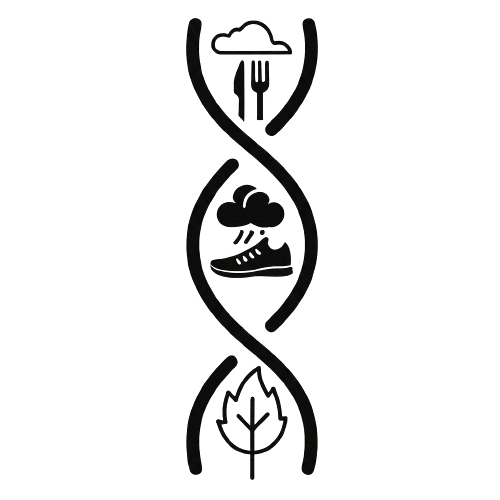
Example of a project centered on genomics and time-varying environments in the Dennis Lab:
Early life adversity and epigenetic aging: Findings from a 17-year longitudinal study
Youth who experience early life adversity (e.g., experiences of peer victimization, growing up low income, and exposure to dysfunctional household dynamics) face worse physical and mental health trajectories in their adolescent and adult years, but we do not know how or why. We hypothesize that DNA methylation (DNAm; a type of epigenetic modification to the DNA) is a mechanism by which early life adversity becomes biologically embedded and ultimately affects later life health outcomes. We are testing this hypothesis using longitudinal data from a population-based cohort of children born in Quebec, Canada. Early life adversity was measured repeatedly from before birth through to 17 years of age, and we are relating these adversity measures to six different measures epigenetic aging. We hope to highlight the unique contributions of early life adversity to our epigenome, to ultimately improve long term health trajectories.